Location Independent Traffic Modeling
Existing transportation models focus on a single location or city, and it is generally painful to build the similar traffic model for another location, even if the model itself is similar. The main challenges are the data availability, data specifications, and practical limitations. In this research topic, we aim to develop location Independent traffic models such that they can be applied and used at different locations in the world. The major technologies we adopted include: transfer learning, representation learning, adversarial machine learning, and multi-source data fusion.
As one example for traffic prediction, we propose a novel transferable traffic forecasting framework: Domain Adversarial Spatial-Temporal Network (DASTNet). DASTNet is pre-trained on multiple source networks and fine-tuned with the target network’s traffic data. Specifically, we leverage the graph representation learning and adversarial domain adaptation techniques to learn the domain-invariant node embeddings, which are further incorporated to model the temporal traffic data. To the best of our knowledge, we are the first to employ adversarial multi-domain adaptation for network-wide traffic forecasting problems. DASTNet consistently outperforms all state-of-the-art baseline methods on three benchmark datasets. The trained DASTNet is applied to Hong Kong’s new traffic detectors, and accurate traffic predictions can be delivered immediately (within one day) when the detector is available. Overall, this study suggests an alternative to enhance the traffic forecasting methods and provides practical implications for cities lacking historical traffic data.
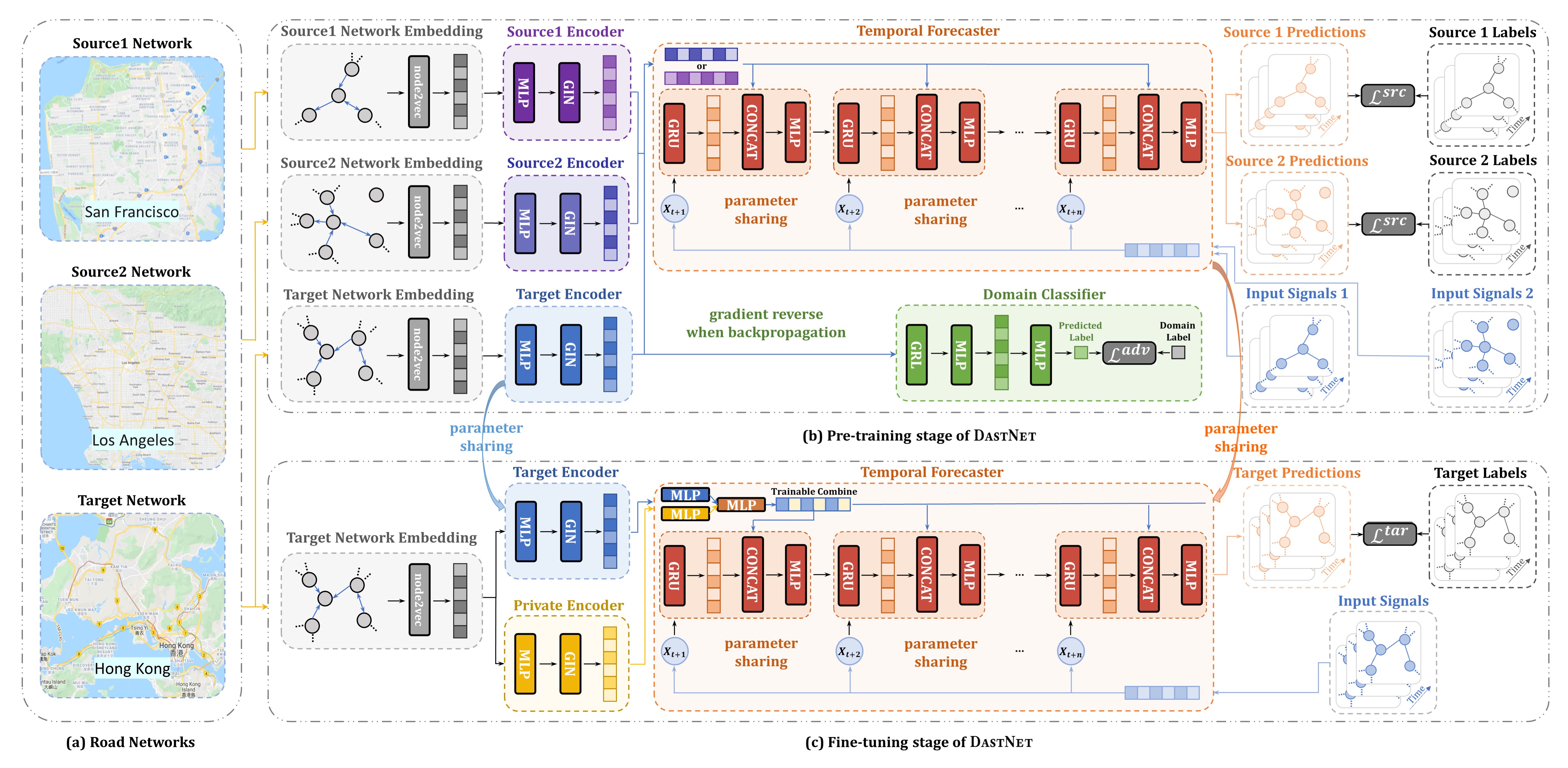