Physics-guided Machine Learning for Infrastructure Systems
Machine Learning (ML) methods are widely used for representation learning, pattern recognition, prediction, classification, and anomaly detection. The ML methods rely on massive and high-quality data, which are usually NOT available in the infrastructure systems. On the contrary, the engineering communities have developed various physical models to depict the activities and inter-connections of different infrastructure systems, such as transportation systems, pipeline systems, building systems, and pavement systems. These physical models include simulations, game theories, (partial) differential equations, statistical models, and tree-based models. How to better train the ML models with knowledge in the physical model presents a great challenge but also opportunity to the research community.
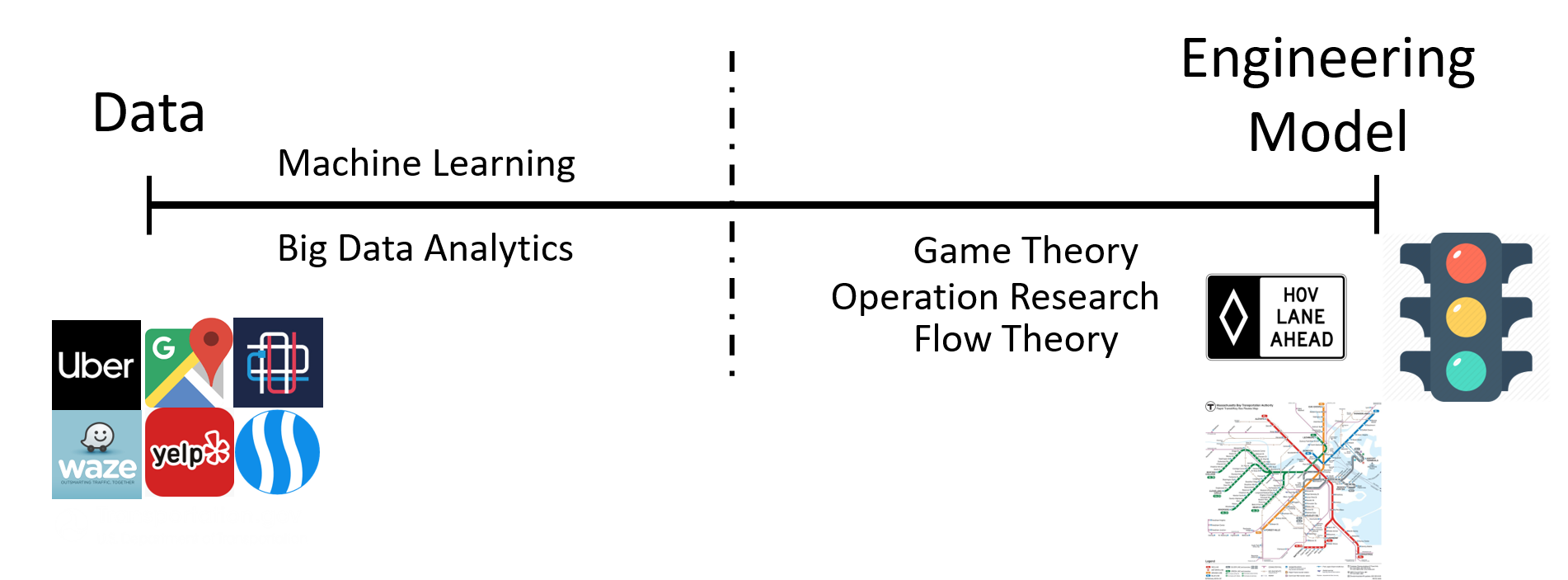