Learning the Dynamics of Transportation Networks through Multi-source Data
With advanced sensing technology and rich data resources, various data sets, including traditional traffic sensors (loops, cameras, etc.) and cutting-edge sensors (Bluetooth, GPS probe, parking, etc.), are available and have been archived for a decade in many mega-cities. However, those data sets are not fully utilized in modeling and decision making for transportation systems. Traffic dynamics of a region/city is still deterministically modeled in by simply taking the average of data over a long time horizon, which leads to deterministic estimation of traffic conditions. The flow variation from time to time (such as morning versus afternoon, today versus yesterday) cannot be captured in those deterministic models. Deterministic traffic models are not friendly to large-scale data, and not suitable for system-wise decision making that largely relies on modeling the flow variation and understanding it causes. With the availability of various traffic data (real-time and historically archived), modeling the stochasticity and uncertainty of traffic dynamics raises a big challenge to public agencies. This research aims at developing a regional dynamic network model that statistically infers millions of trips in the metropolitan regions and captures those travelers’ behavior through massive traffic data from different resources.
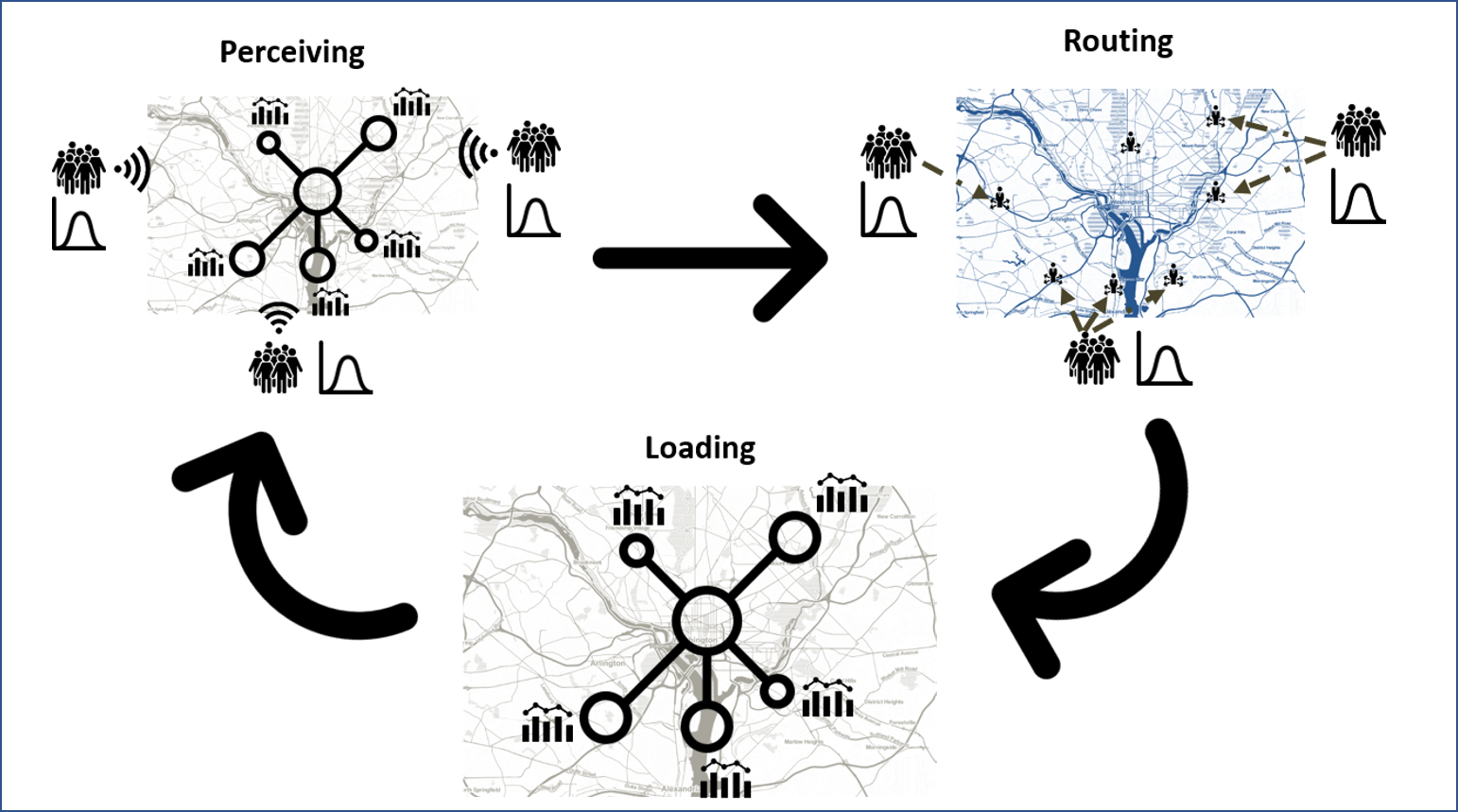